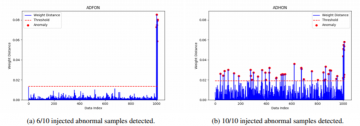
Achievement
We present a new approach based on the higher-order network (HON) representation to detect anomalies in sequential health care data using electronic health records.
Significance and Impact
The results show that there are higher-order dependencies in health care data; and that the use of HON representation is more effective than the first-order network representation for detecting anomalies in sequential health care data.
Research Details
- We used Anomaly Detection based on HON Differences (ADHON) to build network representation of EHR Data.
- We compared ADHON with Anomaly Detection based on First Order Networks (ADFON)
Citation:
Niu, Haoran, Omitaomu, Olufemi, Cao, Qing, Ozmen, Ozgur, Klasky, Hilda, Olama, Mohammed M., Pullum, Laura, Kuruganti, Teja, Ward, Merry, Laurio, Angela, Scott, Jeanie, Drews, Frank, & Nebeker, Jonathan R. Anomaly Detection in Sequential Health Care Data using Higher-Order Network Representation. United States.
Overview
The ever-increasing use of information technology (IT) in health care presents new challenges to patient care. Information errors arising from the use of Health IT and their implications on care delivery and patient outcomes have been widely reported. Information errors can lead to changes in clinical decisions, care processes, and care outcomes, among others. An altered care process, for example, could interrupt the sequence of care, which could lead to changes in care decisions and/or changes in care outcomes. We define interruptions in the care process as anomalies in the care sequence. In this paper, we present a new approach based on the higher-order network (HON) representation to detect anomalies in sequential health care data using electronic health records. The results show that there are higher-order dependencies in health care data; and that the use of HON representation is more effective than the first-order network representation for detecting anomalies in sequential health care data.
Last Updated: March 1, 2021 - 2:24 pm